In our previous blog post we covered the important topic of cybersecurity with a focus on Quantum Security. This blog post continues the #CybersecurityAwarenessMonth theme with a focus on AI Security, covering scenarios including Edge AI and what companies can do to be prepared. Additionally, this post shows that AI is an opportunity in the security field for various scenarios to help with better predictive security analytics and dynamic pattern detection leveraging Machine Learning.
Cybersecurity is an Essential Business Element
In the Quantum Security post we highlighted the importance of privacy and security for business leaders and we mentioned that hacker attacks, breaches, fraud and various other cybersecurity topics are a daily concern with increased attacks in all lines of businesses. This is also the motivation for the shift to Zero Trust models where organizations are not only preparing for worst cases, but are assuming that data breaches and similar things happen all the time. They are designing their systems to be able to handle an appropriate response quickly.
A recent Forrester report highlighted that privacy and data protection are also important for consumers when deciding whether to engage with a company. “62% of US and 55% of UK consumers say that how a company handles their personal information is important to their purchasing decisions.” Therefore, customer data needs to be stored and handled securely to ensure no data breaches happen and attacks are controlled appropriately to also protect customer data which is more and more important to comply with privacy regulations like GDPR and alike. “For firms that report being fully compliant with GDPR, there’s a strong alignment between business and security. Forrester found that these firms protect assets, environments, and systems that the business needs much more than firms with less mature privacy programs. Security decision-makers at firms that must comply with GDPR are more likely to say that they have policies and tools in place to adequately secure the use of AI technologies, blockchain, DevOps, embedded internet of things solutions, and [more].” AI in particular is a key technology for customer-obsessed firms' innovation and transformation strategies. The reason is the amount of customer and employee data available nowadays, and the growth of AI model capabilities that can use that data in the best interest of the customers which implicitly includes the need for the highest level of privacy and cybersecurity methodologies in place like Zero Trust.
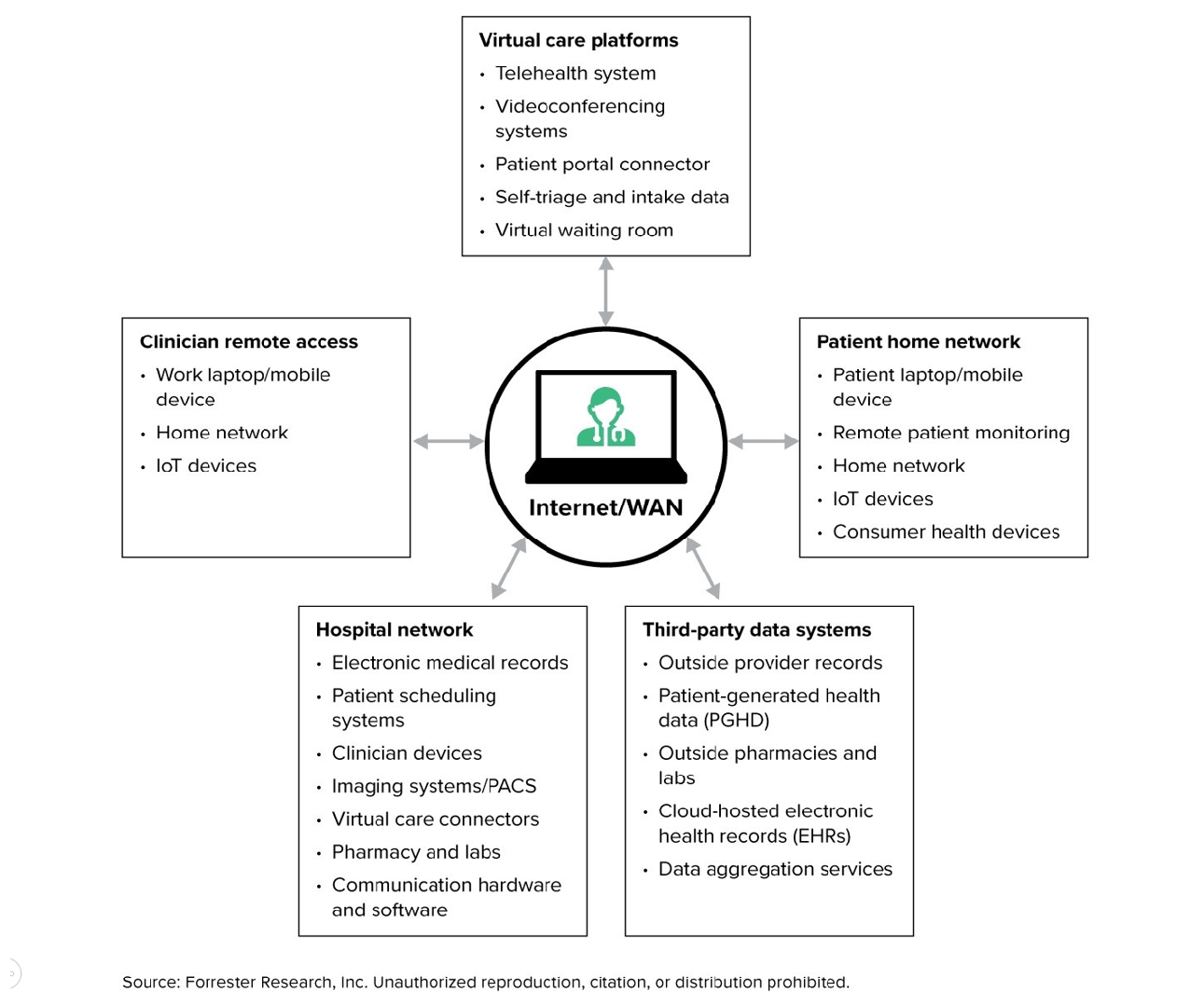
Figure 1: Increasing data flow between internal and external systems puts patient data at risk. (Graphic from Forrester, “The Zero Trust Security Architecture For Healthcare” by Chris Sherman, Alla Valente with Merritt Maxim, David Holmes, Benjamin Corey, Peggy Dostie, June 2021. See https://www.forrester.com/report/The-Zero-Trust-Security-Architecture-For-Healthcare/RES165876 for more information.)
One industry under attack is Healthcare where attacks further accelerated during COVID-19 as Forrester reports. The reasons are mainly due to introduction of virtual health models, acceleration of IoT and remote patient monitoring devices and the expansion of third-party relationships. For example, AI-assisted clinical decision support services integrating with hospital systems running analyses on patient data remotely. Healthcare organizations currently invest in securing protected health information (PHI) but must also invest in strategies securing the entire organization, by implementing a Zero Trust framework that ensures security and privacy holistically.
AI Security is Regular Cybersecurity Plus Specialties
Most security attacks to enterprise systems can also be a risk for AI/ML workloads which implicates that the same threat and security solutions can be applied for AI security, already in-place to protect the enterprise network. Furthermore, AI models and in particular computer vision Deep Learning systems for image recognition, are also vulnerable for malicious users who could access sensitive data to introduce new or altered images for the AI model training which results in errors or bias. Like we wrote in our Digital Ethics and Responsible AI post, enterprise security helps to protect from maliciously modified, biased model training that could contain a non-inclusive, not-diverse dataset, resulting in biased predictions. Bias is already a common problem for certain AI scenarios like Twitter’s photo-cropping algorithm has shown which preferred faces that are “slim, young, of light or warm skin color and smooth skin texture, and with stereotypically feminine facial traits.” Introduction of bias typically does not happen on-purpose, but it could also be done explicitly by a malicious user behind the scenes who is sabotaging training data. AI models are typically opaque black boxes and such a sabotage with training data poisoning might only be noticed much later after training when it’s too late and the AI model is being used in production for predictions.
AI Attacks are Increasing
A paper by the Artificial Intelligence/Machine Learning Risk & Security Working Group (AIRS) of Wharton School identified these and additional AI Risks. For example, model extraction is the most impactful one where an attacker tries to steal the whole AI model with usually very sensitive IP. Model extraction could also lead to more attacks by analyzing the model further for more targeted attacks. AIRS mentions with an unlimited ability to query an AI model, the model extraction could occur at high speeds without the need to have high levels of technical sophistication. This indicates that public APIs that use an AI model under the hood need to be protected against these types of attacks by limiting the query ability with classic API protection mechanisms.
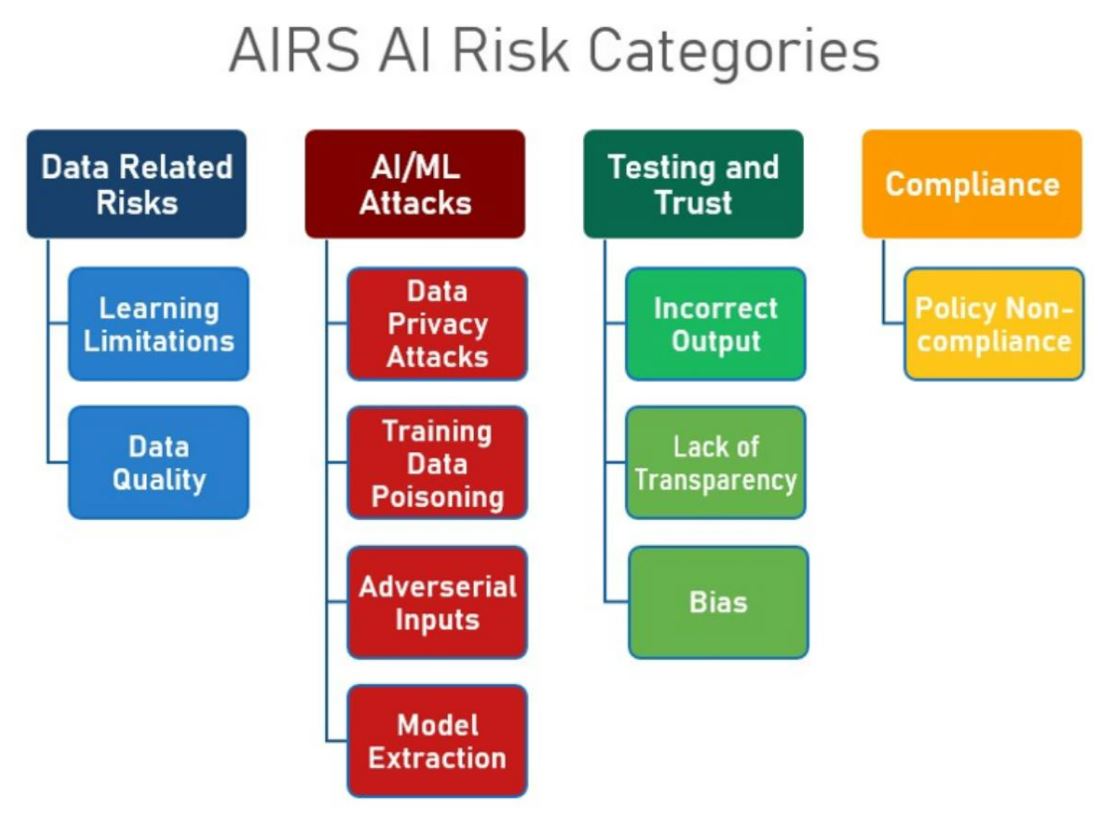
Figure 2: AI Risks and Risk Categorization by AIRS of Wharton School. (Source: https://ai.wharton.upenn.edu/artificial-intelligence-risk-governance)
Additionally, data privacy attacks are highlighted like model inversion and membership inference attacks where an attacker can infer the data that was used to train the AI model which potentially compromises the privacy just by analyzing the parameters or querying the model. Another form of an attack that is also leveraging the AI model in its inference phase is using malicious payload and input to trigger unwanted outputs and actions. One common use case for this adversarial input attack is to trick the computer vision AI used in autonomous vehicles to perform the wrong action via modified traffic signs like it was shown in this paper and more.

Figure 3: Autonomous car with an AI adversarial input attack via a slightly modified traffic sign. (Source: http://adversarial-learning.princeton.edu/darts/)
These kind of attacks increase as Microsoft highlights and also market research firm Gartner predicts that through next year (2022), 30% of all AI cybersecurity attacks will leverage AI model theft, training-data poisoning, or adversarial samples to target AI systems. These risk challenges are one reason why security and privacy concerns for AI deployments are the top blocker for adoption according to another Gartner survey. Additionally, a Microsoft study with 28 organization spanning Fortune 500 companies, SMB, non-profit and government organizations found out that 25 out of the 28 businesses indicated they don’t have the right tools in place to secure their AI systems and their security professionals are looking for guidance in that space. Fortunately there’s also good work being done to fill that gap, for example, Microsoft worked with MITRE to create the so called “Adversarial Threat Landscape for Artificial-Intelligence Systems” (ATLAS). ATLAS is a knowledge base that systematically organizes the attack techniques and gives security teams an interactive tool to navigate the landscape of threats to AI/ML systems. The interactive ATLAS guidance is a good start but Microsoft implemented this knowledge also into the tool Counterfit which is an open-source automation tool for security testing of AI/ML systems. Counterfit helps organizations to conduct AI security risk assessments to ensure their algorithms are reliable, robust, and trustworthy.
If you want to learn more why also ethical usage of AI is essential to counteract the mistrust in AI solutions, help organizations to balance risk and build trust and how to mitigate bias and AI risks further, take a look at our Digital Ethics and Responsible AI post.
Cybersecurity is Moving to the Edge with Edge AI Security
Security is an important topic for intelligent cloud solutions that run AI workloads centralized. Thankfully, all big cloud vendors like Microsoft’s Azure have large, dedicated security teams ensuring short response times and quick security mitigations. This is another benefit of cloud solutions vs. on-premises since the cloud machines are managed and always have the latest security patches supported by teams of experienced security engineers. A recent example is the largest DDoS attack that was ever recorded with 2.4 Terabits per second workload that Microsoft Azure fended off and was able to stay online, even under this massive large-scale attack.
For a modern organization, cloud security is just one part of the strategy since cybersecurity does not only affect networks, (cloud) servers, PCs and people, but also all smartphones, and actually every machine accessing the organization’s environment including IoT devices. Like we mentioned in the Quantum Security post, the Forrester IoT Security Report4 found out that security decision-makers on average indicate that 10% of their security budget will go to IoT Security with a growing spending priority. This shift to decentralized solutions is even stronger with AI and intelligent edge solutions leveraging improved response time and bandwidth reduction with low-latency computation closer to the IoT sensor on the edge. This is a topic we covered in-depth in this Edge AI Video Analytics post where we also presented the below custom Edge AI demo:
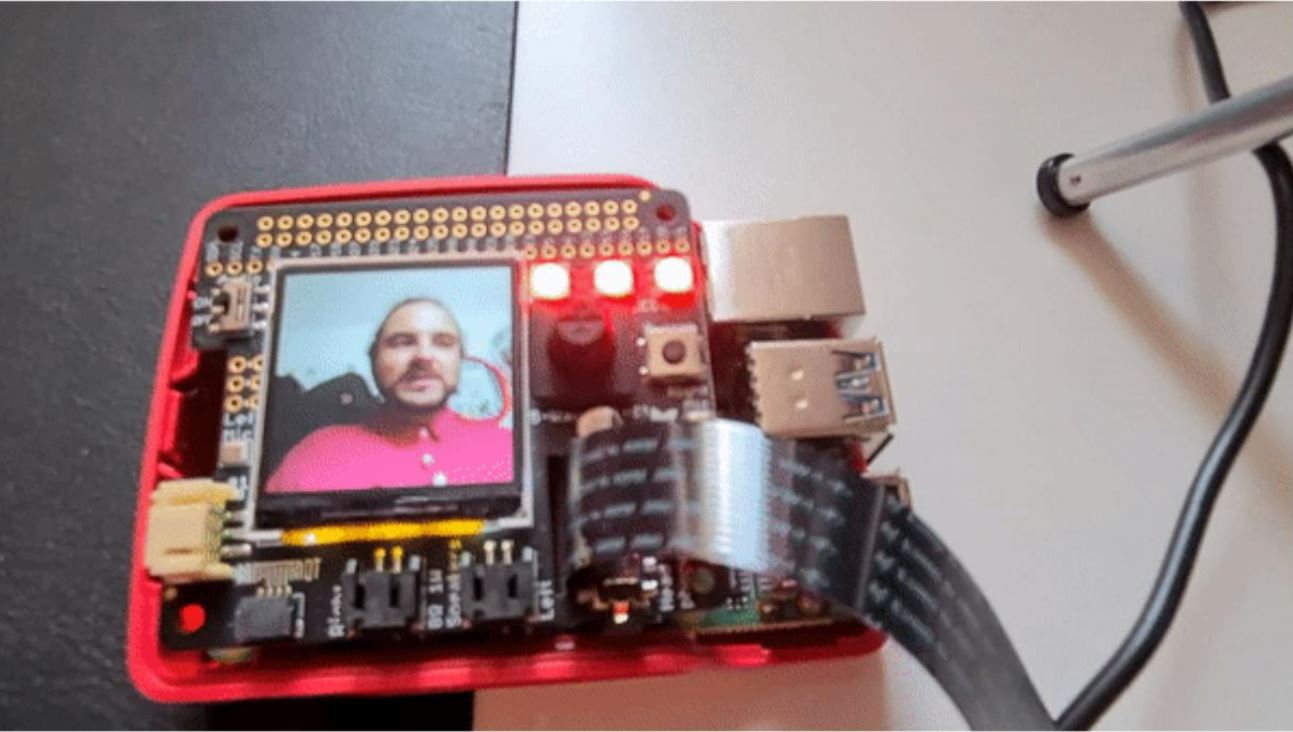
Figure 4: Edge AI Workplace Safety Demo with Adafruit Raspberry Pi from a previous post. The Deep Learning model can detect if the person in front of the camera is wearing “Safety Glasses” or “No Glasses”.
Naturally this shift to edge cloud AI with computing not physically housed in the same data center but distributed out in-the-field also brings higher security risks. Edge deployments don’t have the same physical access model as cloud data centers where physical access to devices is highly controlled. Far more scattered attack entry points exist with edge deployments making Edge AI systems vulnerable for stealing valuable IP and private data. For IoT and other edge deployments, the assumption must be that everyone can potentially get physical access to the edge device. Therefore, Zero Trust is an important paradigm here as well. Edge devices can also be equipped with signed AI container workloads and Trusted Platform Module (TPM) making malicious modifications of firmware and operating system very hard.
AI is an Opportunity for Better Cybersecurity
As much as AI/ML systems are under attack and a security concern, they also provide opportunities to enhance security practices with Machine Learning. One example is fraud management, where ML models trained with valid and malicious transactions can augment accounting auditors to detect fraud faster with high frequency transactions generating lots of transactional data that needs to be analyzed. Additionally, this approach can be used to empower a junior security engineer with the experience of a senior security expert, used to train a ML model which later augments the knowledge of the junior investigator allowing to scale better.
AI/ML is also being used to make automatic pattern detection more robust. For example, when an employee wants to enter a building outside of their typical working hours a classic system would just use a simple Yes/No decision based on a comparison of current time vs. the working hours. An enhanced systems could leverage more historic data via ML and potentially have a better, non-binary decision. For example, the employee might come once a month outside the typical working hours to run tasks like computer updates at night. An AI-enhanced system would have learned that pattern from the historic data and would not trigger an unnecessary alarm therefore saving time and money for the security team. This is just a simple pattern, but AI/ML systems are capable of learning much more complex usage patterns that even humans might not be able to identify.
Complex patterns analysis could also be leveraged for predictive security analytics. Such as, an AI would look at a person’s security enablement and would check what harmful things a person could perform. They could they trigger a payment within the company and at the same time that person has the rights to process gains, allowing to potentially loop transactions. With more and more complex security policies and access layers also on an app-level, it is often not a straight route to check for such conflicting access rights. To check for such issues, could also include having to go down inside the app layer into the business process layer. This is where AI/ML systems can also help with better automatic identification of potential risks and conflicts of interests within an organization.
From a user and identify perspective, conversational AI models like chatbots, can help to reduce the workload of IT departments with solutions like self-service chatbots that allow to reset passwords and perform other user identity actions. Chatbots are a great enabler in general and you can read more about chatbots in this great article by Liji Thomas.
Where to get started?
Valorem and the global Reply network are here to help with our experienced teams of security and AI experts that can meet you where you are on your AI and security journey. If you have a security project in mind or would like to talk about your path to security, we would love to hear about it! Reach out to us as marketing@valorem.com to schedule time with one of our experts.